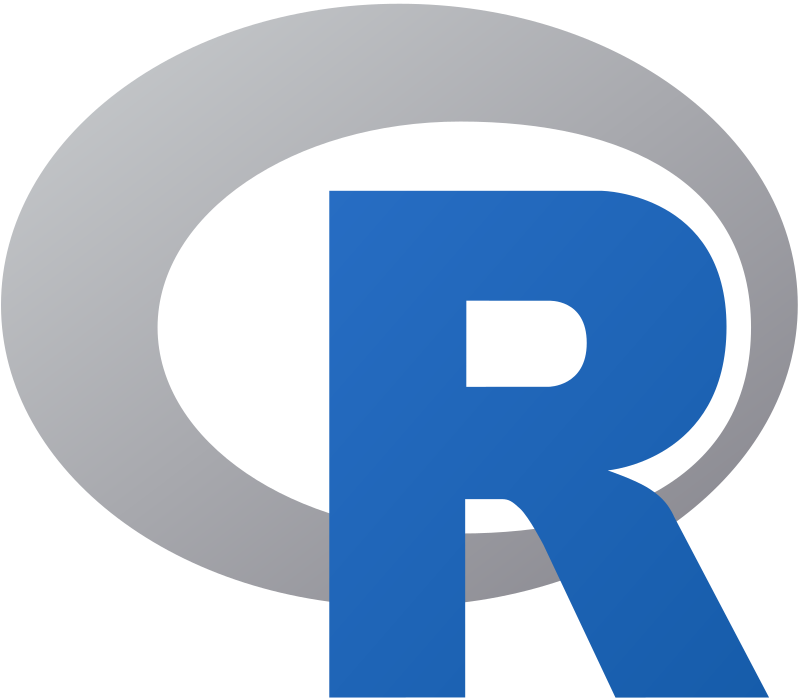
The dplyr
and data.table
part are based on the courses Data Manipulation in R with dplyr and Data Manipulation in R, the data.table way on DataCamp. Hope the description along with the code in this guide help you understand the basic data wrangling in R clearly.
dplyr
Overview
- Use
tbl_df
to convertdata.frame
into data frame table.tbl
is a special type of data.frame. glimpse
(fromtibble
) display more info of the data table. It checks every column in the set, similar to R base functionstr
.- Convert tbl back to data.frame.
as.data.frame(iris)
data('iris')
# change names
names(iris) <- c('SL', 'SW', 'PL', 'PW', 'Species')
# covert the data.frame into tbl
library(dplyr)
iris <- tbl_df(iris)
iris # R only disply a portion of the tbl fits the console window
## # A tibble: 150 <U+00D7> 5
## SL SW PL PW Species
## <dbl> <dbl> <dbl> <dbl> <fctr>
## 1 5.1 3.5 1.4 0.2 setosa
## 2 4.9 3.0 1.4 0.2 setosa
## 3 4.7 3.2 1.3 0.2 setosa
## 4 4.6 3.1 1.5 0.2 setosa
## 5 5.0 3.6 1.4 0.2 setosa
## 6 5.4 3.9 1.7 0.4 setosa
## 7 4.6 3.4 1.4 0.3 setosa
## 8 5.0 3.4 1.5 0.2 setosa
## 9 4.4 2.9 1.4 0.2 setosa
## 10 4.9 3.1 1.5 0.1 setosa
## # ... with 140 more rows
# change column names
names(iris) <- c('SL', 'SW', 'PL', 'PW', 'Species')
# change the level name of factor
new_level <- c('setasa'='set', 'versicolor'='ver', 'virginica'='vir')
iris$new_Species <- as.factor(new_level[iris$Species])
# check more info of data table
glimpse(iris)
## Observations: 150
## Variables: 6
## $ SL <dbl> 5.1, 4.9, 4.7, 4.6, 5.0, 5.4, 4.6, 5.0, 4.4, 4.9, ...
## $ SW <dbl> 3.5, 3.0, 3.2, 3.1, 3.6, 3.9, 3.4, 3.4, 2.9, 3.1, ...
## $ PL <dbl> 1.4, 1.4, 1.3, 1.5, 1.4, 1.7, 1.4, 1.5, 1.4, 1.5, ...
## $ PW <dbl> 0.2, 0.2, 0.2, 0.2, 0.2, 0.4, 0.3, 0.2, 0.2, 0.1, ...
## $ Species <fctr> setosa, setosa, setosa, setosa, setosa, setosa, s...
## $ new_Species <fctr> set, set, set, set, set, set, set, set, set, set,...
Five verbs for data manipulation. select
, filter
, arrange
, mutate
, summarize
.
select()
Select(Remove) the columns by name and return a table
which contains the selected columns. The original table
is not modified. If you want to use the returned table later, save it into a new object. new_table <- select(iris, var1, var2)
.
- The grammer is
select(data, var1, var2)
. We can put variable names into a stringc(var1, var2)
also. - Use colon
:
to select a range of columns. Use-
to drop columns.select(data, var1:var4, -var2)
. select
also works with column index.select(data, 1:4, -2)
- Helper function for selection, check
select_helpers
.starts_with("X")
: every name that starts with"X"
,ends_with("X")
: every name that ends with"X"
,contains("X")
: every name that contains"X"
,matches("X")
: every name that matches"X"
, where"X"
can be a regular expression,num_range("x", 1:5)
: the variables namedx01, x02, x03, x04 and x05
,one_of(x)
: every name that appears in x, which should be a character vector.
select_
accepts string(only) as paramters which used often when programming with select.
select(iris, SL, Species) # select two columns
## # A tibble: 150 <U+00D7> 2
## SL Species
## <dbl> <fctr>
## 1 5.1 setosa
## 2 4.9 setosa
## 3 4.7 setosa
## 4 4.6 setosa
## 5 5.0 setosa
## 6 5.4 setosa
## 7 4.6 setosa
## 8 5.0 setosa
## 9 4.4 setosa
## 10 4.9 setosa
## # ... with 140 more rows
# select(iris, SL:Species, -SW) # select columns between SL and Species except SW
# select(iris, c(1:2, 4:5))
# select(iris, 1:3, -2) # select columns between index 1 and 3 except 2
# select(iris, starts_with('S'), ends_with('L')) # for more than one helper function, the relation is `OR`
mutate()
- Use the data to build new columns and update the original data set.
- The new created column can be referred in later expression.
iris_2 <- mutate(iris, area_S = SL*SW, area_S2 = area_S^2)
head(iris_2)
## # A tibble: 6 <U+00D7> 8
## SL SW PL PW Species new_Species area_S area_S2
## <dbl> <dbl> <dbl> <dbl> <fctr> <fctr> <dbl> <dbl>
## 1 5.1 3.5 1.4 0.2 setosa set 17.85 318.6225
## 2 4.9 3.0 1.4 0.2 setosa set 14.70 216.0900
## 3 4.7 3.2 1.3 0.2 setosa set 15.04 226.2016
## 4 4.6 3.1 1.5 0.2 setosa set 14.26 203.3476
## 5 5.0 3.6 1.4 0.2 setosa set 18.00 324.0000
## 6 5.4 3.9 1.7 0.4 setosa set 21.06 443.5236
filter()
- Remove the rows.
- Use
filter
together with relational operators. CheckComparison
.<, >, <=, >=, ==, !=, %in%
. - For more than one conditions, use boolean operators
&
,|
,!
. filter_
accepts string as parameters as well.
filter(iris, SL == 5.1 & SW >=3.5)
## # A tibble: 6 <U+00D7> 6
## SL SW PL PW Species new_Species
## <dbl> <dbl> <dbl> <dbl> <fctr> <fctr>
## 1 5.1 3.5 1.4 0.2 setosa set
## 2 5.1 3.5 1.4 0.3 setosa set
## 3 5.1 3.8 1.5 0.3 setosa set
## 4 5.1 3.7 1.5 0.4 setosa set
## 5 5.1 3.8 1.9 0.4 setosa set
## 6 5.1 3.8 1.6 0.2 setosa set
arrange()
- Reorder the rows in a data set
- Use
desc()
to arrange in descending order, defaul is ascending. - Support arrange by several variables using comma
,
.
# rearrange the table by SL and SW in ascending order
arrange(iris, SL, SW)
## # A tibble: 150 <U+00D7> 6
## SL SW PL PW Species new_Species
## <dbl> <dbl> <dbl> <dbl> <fctr> <fctr>
## 1 4.3 3.0 1.1 0.1 setosa set
## 2 4.4 2.9 1.4 0.2 setosa set
## 3 4.4 3.0 1.3 0.2 setosa set
## 4 4.4 3.2 1.3 0.2 setosa set
## 5 4.5 2.3 1.3 0.3 setosa set
## 6 4.6 3.1 1.5 0.2 setosa set
## 7 4.6 3.2 1.4 0.2 setosa set
## 8 4.6 3.4 1.4 0.3 setosa set
## 9 4.6 3.6 1.0 0.2 setosa set
## 10 4.7 3.2 1.3 0.2 setosa set
## # ... with 140 more rows
# in descending order
# arrange(iris, SL, desc(SW))
# arrange by sum of SL and SW
# arrange(iris, SL+SW)
summarise()
- Calculate summary statistics.
summarise(tbl, sum=sum(A), avg=mean(B), var=var(B))
- You can use any function in
summarise
as long as the function can take a vector of data and return a single number.min
,max
,mean
,median
,quantile(x, p)
-p is the quantile of vector x,sd
,var
,IQR
-Inter Quartile Range of vector x,diff(range(x))
-total range of vector x. dplyr
aggrgate function.first(x)
,last(x)
,nth(x, n)
-the nth element of vector x.n()
-the number of rows in the data.frame or group of observations thatsummarise()
describes.n_distinct(x)
-the number of unique values in vector x.
summarise(iris, mean_SL=mean(SL), nth_PW=nth(PW, 10), distinct_Species=n_distinct(Species), N=n())
## # A tibble: 1 <U+00D7> 4
## mean_SL nth_PW distinct_Species N
## <dbl> <dbl> <int> <int>
## 1 5.843333 0.1 3 150
pipe operator %>%
- From magrittr package. Effecient, readable, save space in memory. We don't need use nested loop.
object %>% function( , arg2, arg3)
,object
is passed as the first argument in the function, and it is written asobject %>% function(arg2, arg3)
# use pipe operator to mutate, then filter
iris %>%
mutate(area_S = SL*SW) %>%
filter(Species == 'setosa' & SW > 3) %>%
summarise(avg = mean(area_S))
## # A tibble: 1 <U+00D7> 1
## avg
## <dbl>
## 1 17.98048
group_by
- Takes an existing tbl and converts it into grouped tbl where operations are performed by group. Its influence becomes clear when calling
summarise()
on a grouped dataset: summarising statistics are calculated for the different groups separately.
iris %>%
group_by(Species) %>%
summarise(avg_SL = mean(SL, na.rm=TRUE), avg_SW = mean(SW)) %>%
arrange(avg_SW)
## # A tibble: 3 <U+00D7> 3
## Species avg_SL avg_SW
## <fctr> <dbl> <dbl>
## 1 versicolor 5.936 2.770
## 2 virginica 6.588 2.974
## 3 setosa 5.006 3.428
# or we can use:
iris %>%
group_by(Species) %>%
summarise(avg_SL = mean(SL, na.rm=TRUE), avg_SW = mean(SW)) %>%
mutate(rank = rank(avg_SL)) %>%
arrange(rank)
## # A tibble: 3 <U+00D7> 4
## Species avg_SL avg_SW rank
## <fctr> <dbl> <dbl> <dbl>
## 1 setosa 5.006 3.428 1
## 2 versicolor 5.936 2.770 2
## 3 virginica 6.588 2.974 3
dplyr
also works with other data set types likedata.table
,data.frame
.
data.table
Overview
- Think data.frame as a set of columns.
data.table
inherits from data.frame, but reduces programming time and computing time.data.table
isdata.frame
, accepted as adata.frame
by other pacakges. For the packages don't knowdata.table
,DT[...]
is redirected todata.frame
methods for square bracket[...]
. - General form:
DT[i, j, by]
,i
correspondsWHERE
in SQL andj
correspondsSELECT
in SQL. It stands for "Take DT, subset rows using i, then calculate j grouped byby
".
Select rows by argument i
- Comma
,
can be ignored. Fordata.table
,iris[1:3]
equals toiris[1:3, ]
. But fordata.frame
,iris[1:3]
returns error if you forget comma. .N
, a special symbol which contains the number of rows when used inside square brackets.
data('iris')
# change names
names(iris) <- c('SL', 'SW', 'PL', 'PW', 'Species')
# covert data.frame to data.table
library(data.table)
iris <- data.table(iris)
# select rows
iris[1:3] # iris[1:3,] returns the same table.
## SL SW PL PW Species
## 1: 5.1 3.5 1.4 0.2 setosa
## 2: 4.9 3.0 1.4 0.2 setosa
## 3: 4.7 3.2 1.3 0.2 setosa
# select the first and second last row.
iris[c(1, .N-1)]
## SL SW PL PW Species
## 1: 5.1 3.5 1.4 0.2 setosa
## 2: 6.2 3.4 5.4 2.3 virginica
Select columns by argument j
- Select columns by
j
. Putj
into.()
, an alias tolist()
in data.tables and they mean the same.iris[, .(SL, SW)]
. NoteDT[, .(B)]
returns adata.table
, whileDT[, B]
returns a vector, so isDT[['B']]
, note column name contained in quotation. AndDT[, c(B,C)]
returns a vector combined withB
andC
. - Compute on columns.
iris[, .(mean_SL=mean(SL), mean_SW=mean(SW)]
. - The generated multiple columns all return the same length. The length of short item get recycled to match the length of large columns.
iris[, .(SL, mean_SL=mean(SL))
. For all rows, they have the samemean_SL
. - Throw anything into
j
.iris[, plot(SL, SW)]
. - Subsetting data.tables.
DT[1:3, 2:4, with=False]
. Check the argumentwith
. The argument is namedwith
because it determines whether the column index should be evaluated within the frame of the data.table, as it would be when using, e.g., base R'swith()
andwithin()
. Whenwith=FALSE
j
is a character vector of column names or a numeric vector of column positions to select.
# select three columns
iris[1:3, .(SL, PL, SW)]
## SL PL SW
## 1: 5.1 1.4 3.5
## 2: 4.9 1.4 3.0
## 3: 4.7 1.3 3.2
# select two columns together with a generated column
iris[, .(SL, SW, area_S = SL*SW)]
## SL SW area_S
## 1: 5.1 3.5 17.85
## 2: 4.9 3.0 14.70
## 3: 4.7 3.2 15.04
## 4: 4.6 3.1 14.26
## 5: 5.0 3.6 18.00
## ---
## 146: 6.7 3.0 20.10
## 147: 6.3 2.5 15.75
## 148: 6.5 3.0 19.50
## 149: 6.2 3.4 21.08
## 150: 5.9 3.0 17.70
# throw anything into `j`
iris[, {print(head(SW))
hist(SW)
NULL}] # return NULL
## [1] 3.5 3.0 3.2 3.1 3.6 3.9
## NULL
# select column using index. Set with=FALSE.
iris[1:3, 2:4, with=FALSE] # the same as R base. If iris is data.frame. iris[1:3,2:4] returns the same
## SW PL PW
## 1: 3.5 1.4 0.2
## 2: 3.0 1.4 0.2
## 3: 3.2 1.3 0.2
# also we can use column name
iris[1:3, SW:PW, with=FALSE]
## SW PL PW
## 1: 3.5 1.4 0.2
## 2: 3.0 1.4 0.2
## 3: 3.2 1.3 0.2
Doing j
by group
by
can have more than one values.by = .(A, B)
- We can call functions in
by
- Shortcut: if there is only one item in
j
orby
, you can drop the.()
notation.
iris[, .(mean_SL = mean(SL)), by=.(Species)]
## Species mean_SL
## 1: setosa 5.006
## 2: versicolor 5.936
## 3: virginica 6.588
# call function in `by`, and grouping only on a subset
iris[1:100, .(mean_SL = mean(SL)), by=.(substr(Species, 1, 1))] # group by the 1st character of Species
## substr mean_SL
## 1: s 5.006
## 2: v 5.936
# count the number of rows by group
iris[, .(Count = .N), by=.(not_setosa <- Species != 'setosa')]
## not_setosa Count
## 1: FALSE 50
## 2: TRUE 100
# select the last two rows of SL by group
iris[, .(last_two_SL = tail(SL, 2)), by=.(Species)]
## Species last_two_SL
## 1: setosa 5.3
## 2: setosa 5.0
## 3: versicolor 5.1
## 4: versicolor 5.7
## 5: virginica 6.2
## 6: virginica 5.9
Chaining
- Chain two
[]
,[]
together.
# select the last two rows of SL by group
iris[, .(SL), by=.(Species)][, .(last_two_SL = tail(SL,2)), by=.(Species)]
## Species last_two_SL
## 1: setosa 5.3
## 2: setosa 5.0
## 3: versicolor 5.1
## 4: versicolor 5.7
## 5: virginica 6.2
## 6: virginica 5.9
# order by selected column
iris[, .(last_two_SL = tail(SL, 2)), by=.(Species)][order(last_two_SL, decreasing = TRUE)]
## Species last_two_SL
## 1: virginica 6.2
## 2: virginica 5.9
## 3: versicolor 5.7
## 4: setosa 5.3
## 5: versicolor 5.1
## 6: setosa 5.0
Subset of data
- A special built-in variable
.SD
, refers to the subset of the data table for each unique value of theby
argument. .SDcols
specifies the columns ofDT
that are included in.SD
. Use.SDcols
if you want to perform a particular operation on a subset of the columns.
# use lapply to compute the mean of each columns
iris[, lapply(.SD, mean), by = .(Species)]
## Species SL SW PL PW
## 1: setosa 5.006 3.428 1.462 0.246
## 2: versicolor 5.936 2.770 4.260 1.326
## 3: virginica 6.588 2.974 5.552 2.026
# use .SDcols to specify the columns(2nd to 4th) selected for each group.
iris[, lapply(.SD, mean), .SDcols = c(2:4), by = .(Species)]
## Species SW PL PW
## 1: setosa 3.428 1.462 0.246
## 2: versicolor 2.770 4.260 1.326
## 3: virginica 2.974 5.552 2.026
# we can also set the value of .SDcols as column names. e.g. .SDcols = c('SL', 'SW')
iris[, lapply(.SD, mean), .SDcols = c('SL', 'SW'), by = .(Species)] # note add quotation ""
## Species SL SW
## 1: setosa 5.006 3.428
## 2: versicolor 5.936 2.770
## 3: virginica 6.588 2.974
Using :=
in j
- Updates the data table by reference.
- Different from selecting by
i
andj
, this is updating data table, and the new data table isn't printed. - Remove columns using
:= NULL
. Remove the columns instantly no matter how large the data is in RAM. The columns can be indicated by both name or column index number. - Functional
:=
.DT[,
:=(y=6:10, )
:=
combined withi
andby
. Note ifi
is set a value, then only those rows are used to compute in the:=
by groups. It doesn't mean selecti
rows in the updated data table. See example in code chunk below.
# reverse a column, create a new column
iris[, c('rev_SL', 'area_S') := .(rev(SL), SL*SW)]
# remove a column. If only one column, there is shortcut: iris[, area_S := NULL]
iris[, c('rev_SL', 'area_S') := NULL]
# if the columns need be deleted is a vector, we need add bracket to stop the variable being a symbol.
# Then data.table knows to look up the value of the variable.
# Also, you can paste
# MyCols <- c('rev_SL', 'area_S')
# iris[, (MyCols) := NULL]
# Functional :=
iris[, `:=`(x = 6:10, # space of comment for each variable, make it easy to read
z = 1)]
# := combined with i and by
# only the 1st 100 rows are selected to compute mean of SL by Species. The `mean_SL` in rows later than 100 are NA
iris[1:100, mean_SL:=mean(SL), by=.(Species)]
iris[c(1:3, 101:103)]
## SL SW PL PW Species x z mean_SL
## 1: 5.1 3.5 1.4 0.2 setosa 6 1 5.006
## 2: 4.9 3.0 1.4 0.2 setosa 7 1 5.006
## 3: 4.7 3.2 1.3 0.2 setosa 8 1 5.006
## 4: 6.3 3.3 6.0 2.5 virginica 6 1 NA
## 5: 5.8 2.7 5.1 1.9 virginica 7 1 NA
## 6: 7.1 3.0 5.9 2.1 virginica 8 1 NA
# an error for deleting columns
iris[, c('x') := NULL] # not error is given if i is specified.
iris[, 6 := NULL] # remove column by number also works.
Use set()
- Assignment by reference, used to repeatedly update a data.table by reference. You can think of the
set()
as loopable, low overhead version of the:=
operator, except thatset()
cannot be used for grouping operations.set(DT, index, column, value)
# randomly set three items in 6th column to be NA
temp <- iris[1:6]
for(i in 6:6) set(temp, sample.int(nrow(temp), 3), i, NA)
temp
## SL SW PL PW Species mean_SL
## 1: 5.1 3.5 1.4 0.2 setosa NA
## 2: 4.9 3.0 1.4 0.2 setosa 5.006
## 3: 4.7 3.2 1.3 0.2 setosa NA
## 4: 4.6 3.1 1.5 0.2 setosa 5.006
## 5: 5.0 3.6 1.4 0.2 setosa 5.006
## 6: 5.4 3.9 1.7 0.4 setosa NA
Use setnames()
, setcolorder
- Use
setnames()
to set or change column names; usesetcolorder()
to reorder the columns.
setnames(temp, names(temp), paste0(names(temp), '_new'))
temp[1]
## SL_new SW_new PL_new PW_new Species_new mean_SL_new
## 1: 5.1 3.5 1.4 0.2 setosa NA
Indexing
- Automatic indexing. Data table don't actually vector scan. They create an index automatically on
SL
the first time you use columnSL
. So second time it's much faster as the index alread exists. In data base, you need create the index by hand, while indata.table
it's done automatically.
head(iris[SL >0 & SL <10 & !Species %in% c('setosa')])
## SL SW PL PW Species mean_SL
## 1: 7.0 3.2 4.7 1.4 versicolor 5.936
## 2: 6.4 3.2 4.5 1.5 versicolor 5.936
## 3: 6.9 3.1 4.9 1.5 versicolor 5.936
## 4: 5.5 2.3 4.0 1.3 versicolor 5.936
## 5: 6.5 2.8 4.6 1.5 versicolor 5.936
## 6: 5.7 2.8 4.5 1.3 versicolor 5.936
iris[SL >1 & SL <11] # second time much faster.
## SL SW PL PW Species mean_SL
## 1: 5.1 3.5 1.4 0.2 setosa 5.006
## 2: 4.9 3.0 1.4 0.2 setosa 5.006
## 3: 4.7 3.2 1.3 0.2 setosa 5.006
## 4: 4.6 3.1 1.5 0.2 setosa 5.006
## 5: 5.0 3.6 1.4 0.2 setosa 5.006
## ---
## 146: 6.7 3.0 5.2 2.3 virginica NA
## 147: 6.3 2.5 5.0 1.9 virginica NA
## 148: 6.5 3.0 5.2 2.0 virginica NA
## 149: 6.2 3.4 5.4 2.3 virginica NA
## 150: 5.9 3.0 5.1 1.8 virginica NA
# remove the `_new` suffix
setnames(temp, names(temp), gsub('\\_new$', '', names(temp))) # `^` means begin with, `$` means end with.
temp[1]
## SL SW PL PW Species mean_SL
## 1: 5.1 3.5 1.4 0.2 setosa NA
# remove the columns end with `_SL`
temp[, names(temp)[grepl('\\_SL$', names(temp))] := NULL]
temp[1]
## SL SW PL PW Species
## 1: 5.1 3.5 1.4 0.2 setosa
Keys
- Set keys on columns,
setkey(DT, A)
, then useDT['b']
to filter the rows whereA == 'b'
. (effectively filter). To data.frame, it turns to error. - Two-column key
setkey(DT, A, B)
. The second column is sorted within each group of the first column by = .EACHI
, allows to group by each subset of knows groups ini
. A key needs to be set prior to useby = .EACHI
.
setkey(iris, Species)
head(iris['setosa'])
## SL SW PL PW Species mean_SL
## 1: 5.1 3.5 1.4 0.2 setosa 5.006
## 2: 4.9 3.0 1.4 0.2 setosa 5.006
## 3: 4.7 3.2 1.3 0.2 setosa 5.006
## 4: 4.6 3.1 1.5 0.2 setosa 5.006
## 5: 5.0 3.6 1.4 0.2 setosa 5.006
## 6: 5.4 3.9 1.7 0.4 setosa 5.006
iris['setosa', mult = 'first'] # 'last' returns the last row
## SL SW PL PW Species mean_SL
## 1: 5.1 3.5 1.4 0.2 setosa 5.006
# nomatch
iris['otherSpecies', nomatch = NA] # default
## SL SW PL PW Species mean_SL
## 1: NA NA NA NA otherSpecies NA
iris['otherSpecies', nomatch = 0]
## Empty data.table (0 rows) of 6 cols: SL,SW,PL,PW,Species,mean_SL
# set two-column key. The second column is sorted within each group of the first column
setkey(iris, Species, SW)
head(iris)
## SL SW PL PW Species mean_SL
## 1: 4.5 2.3 1.3 0.3 setosa 5.006
## 2: 4.4 2.9 1.4 0.2 setosa 5.006
## 3: 4.9 3.0 1.4 0.2 setosa 5.006
## 4: 4.8 3.0 1.4 0.1 setosa 5.006
## 5: 4.3 3.0 1.1 0.1 setosa 5.006
## 6: 5.0 3.0 1.6 0.2 setosa 5.006
iris[.('setosa', 3)]
## SL SW PL PW Species mean_SL
## 1: 4.9 3 1.4 0.2 setosa 5.006
## 2: 4.8 3 1.4 0.1 setosa 5.006
## 3: 4.3 3 1.1 0.1 setosa 5.006
## 4: 5.0 3 1.6 0.2 setosa 5.006
## 5: 4.4 3 1.3 0.2 setosa 5.006
## 6: 4.8 3 1.4 0.3 setosa 5.006
# first and last row of the `setosa` group
iris[c('setosa'), .SD[c(1, .N)], by=.EACHI]
## Species SL SW PL PW mean_SL
## 1: setosa 4.5 2.3 1.3 0.3 5.006
## 2: setosa 5.7 4.4 1.5 0.4 5.006
Rolling joins
- The value we are looking for falls in a gap in the ordered series in a key. See more details here. It's often used in time series data.
DT <- data.table(A= letters[1:3], B=1:6, C=6:1)
setkey(DT, A, B)
DT
## A B C
## 1: a 1 6
## 2: a 4 3
## 3: b 2 5
## 4: b 5 2
## 5: c 3 4
## 6: c 6 1
DT[.('b', 4)] # C is NA
## A B C
## 1: b 4 NA
DT[.('b', 4), roll = TRUE] # C is 5
## A B C
## 1: b 4 5
DT[.('b', 4), roll = 'nearest'] # C is 2
## A B C
## 1: b 4 2
DT[.('b', 4), roll = +Inf]
## A B C
## 1: b 4 5
DT[.('b', 4), roll = -Inf]
## A B C
## 1: b 4 2
DT[.('b', 4), roll = TRUE, rollends = FALSE]
## A B C
## 1: b 4 5
R build-in data.frame
Overview of data.frame
data('iris')
# change names
names(iris) <- c('SL', 'SW', 'PL', 'PW', 'Species')
str(iris)
## 'data.frame': 150 obs. of 5 variables:
## $ SL : num 5.1 4.9 4.7 4.6 5 5.4 4.6 5 4.4 4.9 ...
## $ SW : num 3.5 3 3.2 3.1 3.6 3.9 3.4 3.4 2.9 3.1 ...
## $ PL : num 1.4 1.4 1.3 1.5 1.4 1.7 1.4 1.5 1.4 1.5 ...
## $ PW : num 0.2 0.2 0.2 0.2 0.2 0.4 0.3 0.2 0.2 0.1 ...
## $ Species: Factor w/ 3 levels "setosa","versicolor",..: 1 1 1 1 1 1 1 1 1 1 ...
Subset a data.frame
- Dollar sign
$
.iris$SL
returns a vector, so isiris[, 'SL']
. iris['SL']
returns one column as data.frame
# select two columns and return data.frame
iris[c('SL', 'SW')][1,]
## SL SW
## 1 5.1 3.5
# use subset()
subset(iris, select = c('SL', 'PL'))[1,]
## SL PL
## 1 5.1 1.4
# use index along with relational operators
iris[iris$SL > 7 & !is.na(iris$Species), 1:3][1, ]
## SL SW PL
## 103 7.1 3 5.9
# use `-` to delete columns by column index. Both with or without a comma work
iris[-5][1, ]
## SL SW PL PW
## 1 5.1 3.5 1.4 0.2
iris[, -5][1, ]
## SL SW PL PW
## 1 5.1 3.5 1.4 0.2
# other expressions to delete columns
# delCols <- 'Species'
# iris[! names(iris) %in% delCols][1, ]
# iris[, ! names(iris) %in% delCols][1, ]
# iris[ -which(names(iris) %in% delCols)][1, ]
# iris[, -which(names(iris) %in% delCols)][1, ]
Add a column
iris$is_setosa <- iris$Species == 'setosa'
iris[1, ]
## SL SW PL PW Species is_setosa
## 1 5.1 3.5 1.4 0.2 setosa TRUE
Combine R object by rows or columns.
# combine by columns. Note the short item is recycled to match the length of large columns.
cbind(iris, z=1)[1, ]
## SL SW PL PW Species is_setosa z
## 1 5.1 3.5 1.4 0.2 setosa TRUE 1
Sort data.frame by columns
- Use function
order
. The return oforder()
are the indices of the ordered vector.
# an example for order. the return of order() are the indices of the ordered vector
# as below, in order(s), 1 is the index of first number(1) of ordered vector(1 2 4 5 7) in original vector.
# 5 is the index of second number(2) of ordered vector(1 2 4 5 7) in original vector, which is 5th.
s <- c(1,5,7,4,2)
sort(s) # [1] 1 2 4 5 7
## [1] 1 2 4 5 7
order(s) # [1] 1 5 4 2 3
## [1] 1 5 4 2 3
s[order(s)] # [1] 1 5 4 2 3
## [1] 1 2 4 5 7
# sort data.frame by multiple columns
head(iris[order(iris$SL, -iris$SW), ])
## SL SW PL PW Species is_setosa
## 14 4.3 3.0 1.1 0.1 setosa TRUE
## 43 4.4 3.2 1.3 0.2 setosa TRUE
## 39 4.4 3.0 1.3 0.2 setosa TRUE
## 9 4.4 2.9 1.4 0.2 setosa TRUE
## 42 4.5 2.3 1.3 0.3 setosa TRUE
## 23 4.6 3.6 1.0 0.2 setosa TRUE
Comments
comments powered by Disqus